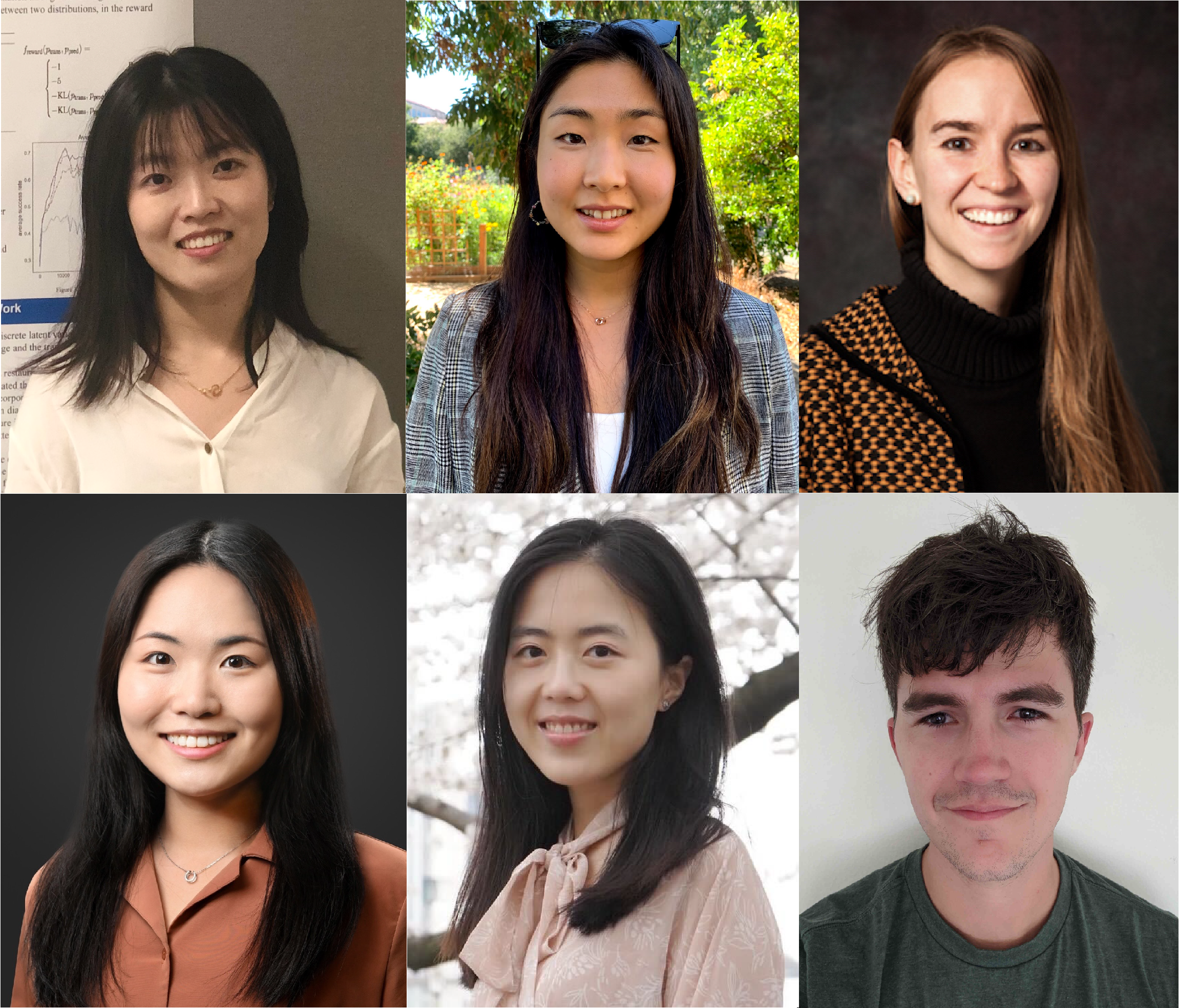
The University of Maryland Center for Machine Learning has selected six winners for its Rising Stars in Machine Learning program—the largest cohort yet.
Now in its fourth year, the program aims to support researchers from underrepresented groups as they pursue new scientific discoveries and academic opportunities. The winners each receive a $1,000 honorarium and the opportunity to present their research at the center’s distinguished seminar series this fall.
“We had a record number of 35 applicants, and with so many having impressive backgrounds and research, it was too difficult to select only three,” says Soheil Feizi, an assistant professor of computer science and leading organizer of the program.
This led Feizi and the center’s interim director, Associate Professor of Computer Science Tom Goldstein, to identify six winners for the first time in the program’s history. Five of this year’s winners identify as women, and one identifies as LGTBQIA+.
The Rising Stars in Machine Learning Seminar Series will kick off on Monday, October 31 with Boyi Li, a research scientist in the machine learning group at NVIDIA and a visiting scholar at the University of California, Berkeley. Her research focuses on multimodal learning, common sense reasoning and human-computer interaction.
At her talk, Li will introduce LSeg, a novel multimodal modeling method for language-driven semantic image segmentation. LSeg uses a text encoder to compute embeddings of descriptive input labels like “grass” or “building” together with a transformer-based image encoder that computes dense per-pixel embeddings of the input image. Her paper shows that LSeg can generalize to previously unseen categories at test time, without retraining or even requiring a single additional training sample.
For more details on Li’s upcoming talk “Learning With and Beyond Visual Knowledge,” subscribe to the University of Maryland Center for Machine Learning email list.
The following day, on November 1, Amanda Coston, a fifth-year Ph.D. candidate in machine learning and public policy at Carnegie Mellon University, will give her talk "Principled Machine Learning for Societally Consequential Decision Making."
Coston uses machine learning to improve decision-making in high-stakes societal settings, specializing in how to make these systems more reliable and equitable. A central focus of her research is identifying when algorithms, data used for policy-making, and human decisions disproportionately impact marginalized groups.
This year’s other winners include Kristy Choi, a fifth-year Ph.D. candidate in computer science and machine learning at Stanford University, whose research is focused on developing techniques for better adaptation and controllability in deep generative models. She grounds methodological work in societal applications motivated by problems in sustainability and fairness.
Wenshuo Guo, a fourth-year Ph.D. candidate in computer science at the University of California, Berkeley, says that her research interests lie at the intersection of machine learning, statistics and economics. In particular, she likes building machine learning systems that are more robust, equitable, and responsive to the evolving social and economic contexts.
Weiyan Shi, a fifth-year Ph.D. candidate in computer science at Columbia University, is focused on natural language processing—especially dialogue systems. Before starting her Ph.D., Shi spent two years working on customer service chatbots as a data scientist at Meta.
Spencer Frei is a postdoctoral fellow at the University of California, Berkeley’s Simons Institute for the Theory of Computing, whose research focuses on the theory and practice of deep learning, non-convex optimization, and statistical learning theory. Last year he graduated with a Ph.D. in statistics from the University of California, Los Angeles.
—Story by Maria Herd