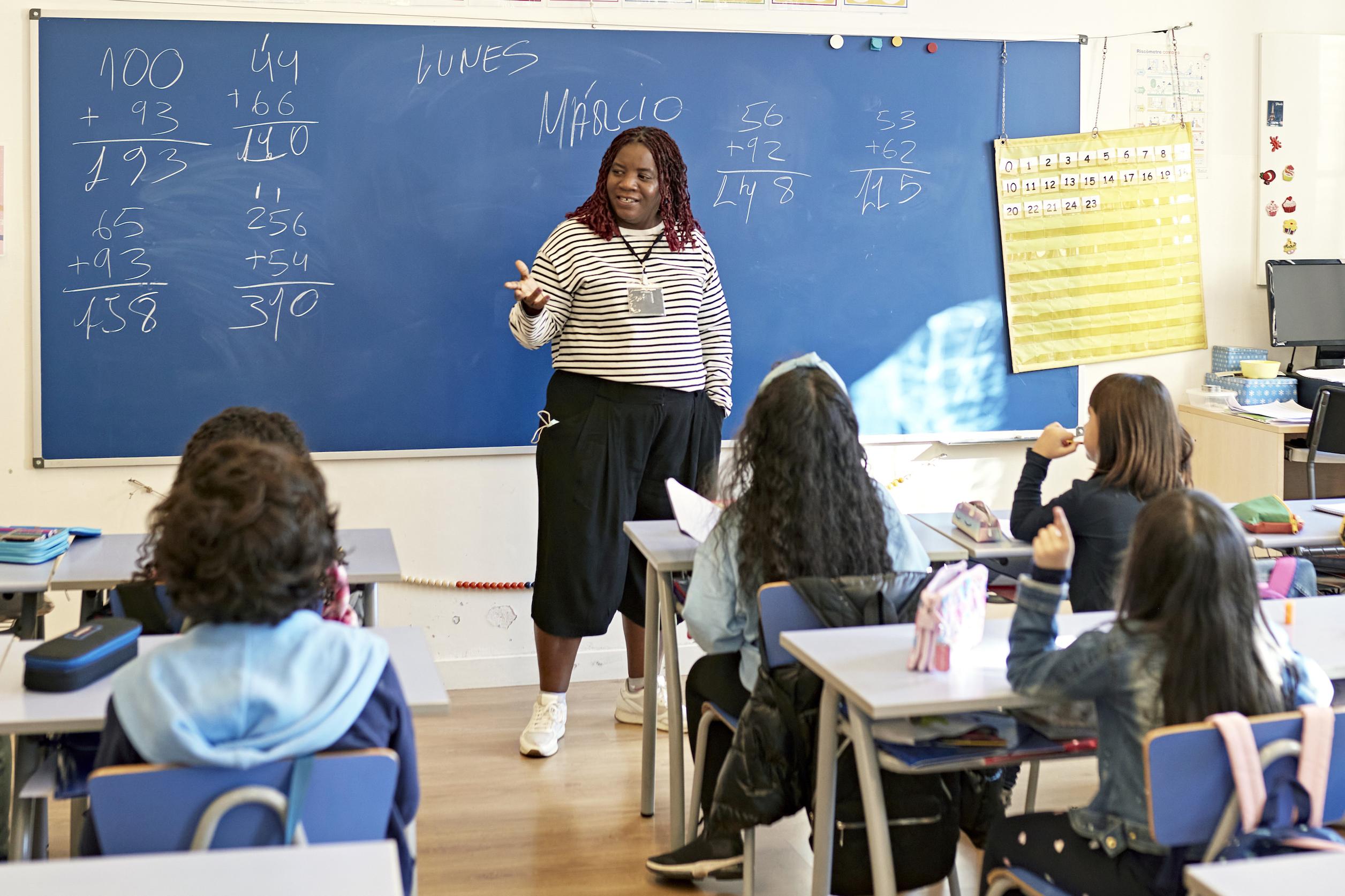
Racial and ethnic disparities in academic achievement are a problematic feature of U.S. education systems, and they remain substantial despite decades of effort.
Academic experts agree that identifying, measuring and improving teaching practices that promote equity is key to creating successful learning contexts that can better serve groups of students who have been historically marginalized.
Wei Ai, an assistant professor in the College of Information Studies, believes one potentially transformative solution to measure and promote equity-centered instruction is through machine learning, a branch of artificial intelligence (AI) and computer science that focuses on the use of data and algorithms to imitate the way that humans learn, gradually improving its accuracy.
He is helping develop an automated feedback platform that will analyze teachers’ instructional moves during class, use state-of-the-art machine learning techniques to quickly assess their skills, and provide them with immediate, ongoing feedback to improve their instruction.
Ai, who also has an appointment in the University of Maryland Institute for Advanced Computer Studies (UMIACS), is collaborating on the project with Carol Espy-Wilson, a professor of electrical and computer engineering; and Jing Liu, an assistant professor in the Department of Teaching and Learning, Policy and Leadership. The research trio was recently awarded a $1 million Team Project Grant to support their work.
Their award comes from UMD’s Grand Challenges Grants Program, a $30 million initiative to support research that offers promising solutions to emerging societal issues like climate change, social injustice, global health and education disparities.
Building on Liu’s recent project that won a global education technology award, this project combines cutting-edge machine learning techniques, rich educational theory, and behavioral sciences to deliver an effective, affordable and scalable mechanism to measure and improve equity-focused teaching practices in K-12 mathematics classrooms.
Liu’s expertise in education policy will help them develop the necessary theoretical framework to assess the types of equitable approaches that should be encouraged in classrooms.
The researchers will rely on Espy-Wilson’s expertise in automated speech recognition to gather recordings and transcriptions of classrooms that are accurate and unbiased.
Ai will bring his expertise in AI and machine learning to synthesize the behavioral data they collect, connect that to educational theories to build the right kind of automated measurements, and then develop theory-guided automated feedback that encourages equity-focused teaching practices.
He explains that their long-term goal is to set up a system that can collect frequent, objective information about a teacher’s practice, and identify strengths and targets for continued improvement.
Ai adds that his team will face challenges such as collaborating with teachers so that they do not feel like they are being monitored or judged, and advancing the automated speech recognition to differentiate young voices in a noisy classroom setting.
Their initial steps include collecting high-quality data and recordings of fourth through sixth grade classrooms, potentially in Los Angeles Unified School District, San Francisco Unified School District, and Prince George's County Public Schools.
“Improving math skills is a national priority, and basis for STEM education, so it’s a natural thing to focus on,” says Liu. “But we are starting to expand its application to the English language arts, and it could be useful for many subjects and disciplines in the future.”
—Story by Melissa Brachfeld, UMIACS communications group