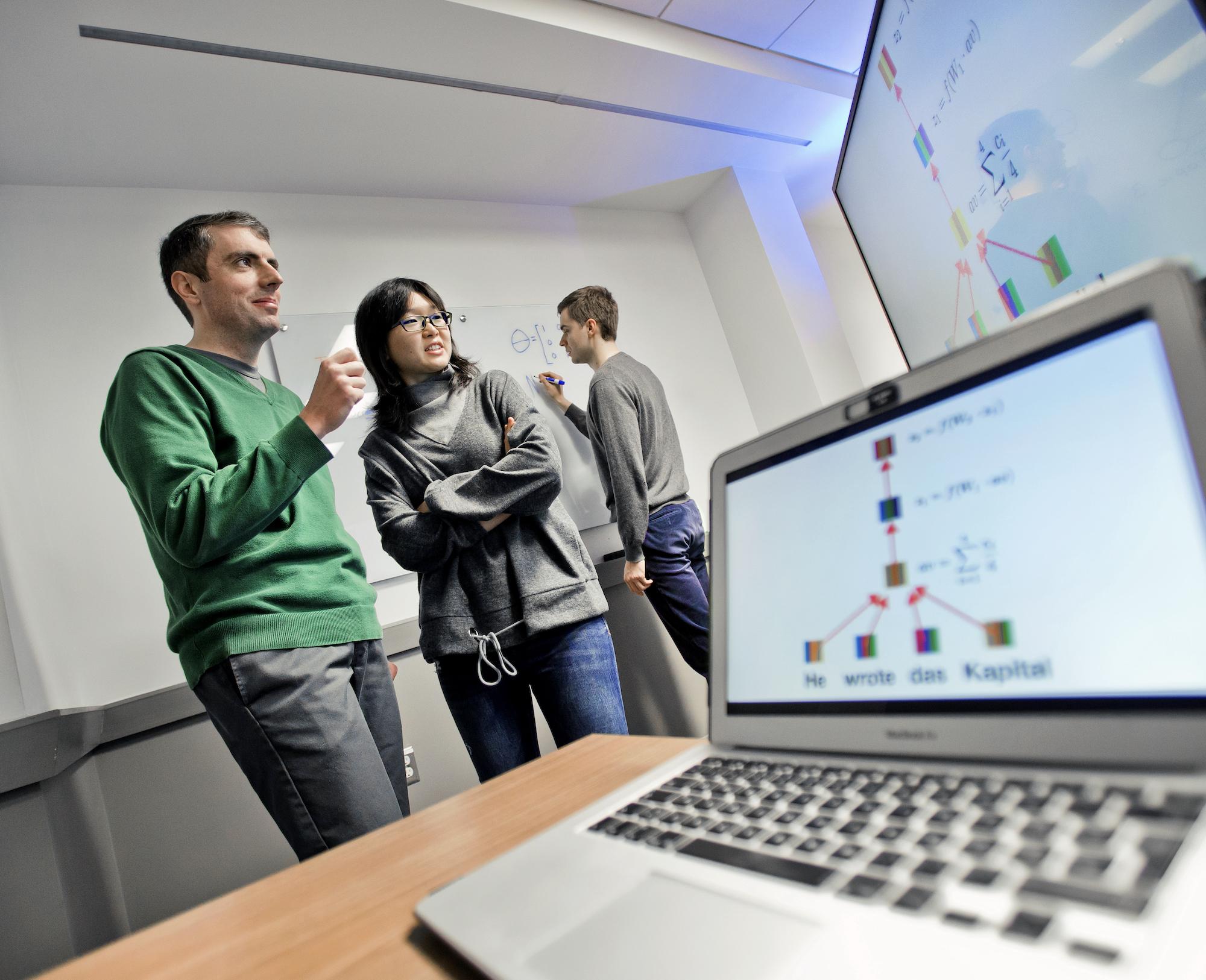
Topic modeling—a machine learning technique originally developed as a text mining tool for computer scientists—is now widely used by historians, journalists and analysts to make sense of large collections of text. These probabilistic models produce various lists of related words, and each list corresponds to a subject in the collection.
But despite their popularity, there are flaws in the way that topic models are evaluated for their accuracy, which ultimately affects how useful they are to the people that rely on them.
In a paper being presented this week at the Conference on Neural Information Processing Systems (NeurIPS), researchers affiliated with the University of Maryland’s Computational Linguistics and Processing (CLIP) Lab argue that topic model developers should reassess the increasing use of machine learning to evaluate their work, and instead revert to a combination of artificial intelligence tools coupled with human input.
“Is Automated Topic Model Evaluation Broken? The Incoherence of Coherence,” closely examines how topic models are automatically evaluated via machine learning algorithms and identifies quirks that can mislead users on their level of quality.
For example, a topic model of the arts in The New York Times may consist of “museum, painting, sculpture, exhibit.” This output should be rated as more coherent than a list like “museum, painting, waffle, piglet,” but that’s not always the case, explains Alexander Hoyle, a third-year doctoral student and the paper’s lead author.
“Our work shows that existing [automated] metrics have severe flaws, even though topic model developers have been relying on them for a long time,” he says.
The CLIP Lab paper was selected as a spotlight presentation for NeurIPS, a recognition given to only three percent of the 9,000 submitted papers. The conference, which takes place virtually December 7–10, is considered the premier gathering for researchers interested in topics at the intersection of machine learning and computational neuroscience.
“The selection of our paper shows that the field is taking the validation of its methods seriously,” says Hoyle. “It also speaks to the rigor of our experimental design and the clarity of our argument.”
Hoyle’s co-authors are Pranav Goel, a fourth-year doctoral student; Denis Peskov, a sixth-year doctoral student; Andrew Hian-Cheong, who received his master’s degree in computer science last year; Jordan Boyd-Graber, an associate professor of computer science with appointments in the University of Maryland Institute for Advanced Computer Studies (UMIACS), the iSchool and the Language Science Center; and Philip Resnik, a professor of linguistics with a joint appointment in UMIACS.
The authors say that their current research builds on prior work, “Reading Tea Leaves: How Humans Interpret Topic Models,” which was co-authored by Boyd-Graber and researchers from Facebook and Princeton University in 2009. That paper argued for measures that correlate with human judgements of quality, but in the decade since the paper, the community had adopted automatic measures that fall short of the human gold standard.
Go here to watch a video presentation of the team’s most recent research on topic modeling.
—Story by Melissa Brachfeld