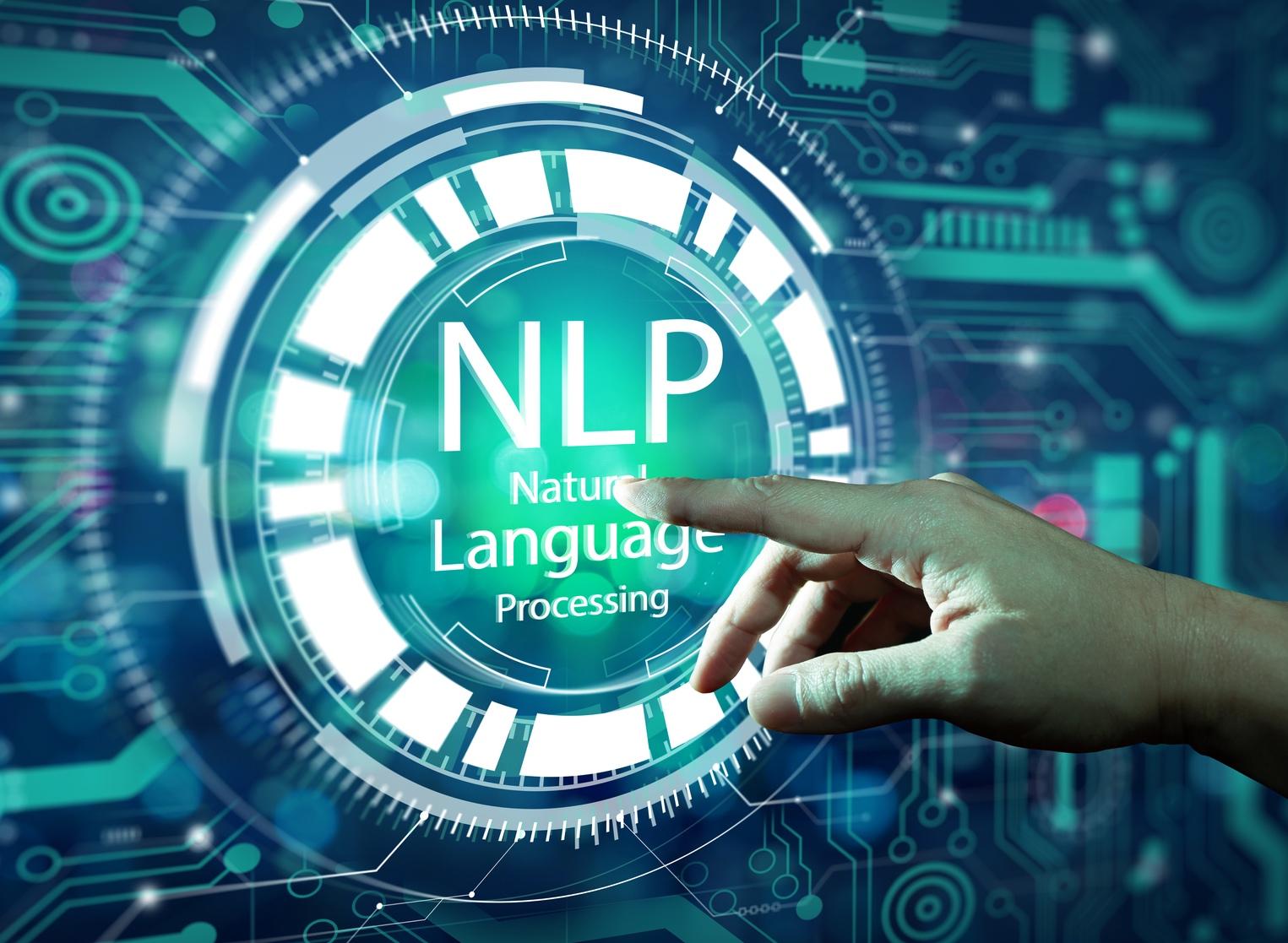
Researchers from the Computational Linguistics and Information Processing (CLIP) Lab are presenting five papers and contributing to several workshops at a top-tier conference for natural language processing this week in Seattle.
The 2022 Annual Conference of the North American Chapter of the Association for Computational Linguistics (NAACL) is being held July 10–15 and streamed online in a hybrid format. CLIP faculty, graduate students, postdoctoral researchers, and alumni are all represented in the conference program.
Marine Carpuat, an associate professor of computer science and program co-chair for this year’s conference, helped come up with the event’s special theme, human-centered natural language processing (NLP).
“As NLP applications increasingly mediate people’s lives, it is crucial to understand how the design decisions made throughout the NLP research and development lifecycle impact people, whether they are users, developers, data providers or other stakeholders,” she says. “For NAACL 2022, we invited submissions that address research questions that meaningfully incorporate stakeholders in the design, development and evaluation of NLP resources, models and systems.”
The research community is increasingly interested in providing explanations of NLP models to help people make sense of model behavior and potentially improve human-interaction. To help address this, Jordan Boyd-Graber, director of CLIP and an associate professor of computer science, is co-leading the tutorial “Human-Centered Evaluation of Explanations.”
Boyd-Graber will also give an invited talk at the First Workshop on Dynamic Adversarial Data Collection. In “Incentives for Experts to Create Adversarial QA and Fact-Checking Examples,” he will discuss two examples of his team’s work putting experienced writers in front of a retrieval-driven adversarial authoring system: question writing and fact-checking.
At the workshop Wordplay: When Language Meets Games, Professor of Computer Science Hal Daumé III will give the invited talk “Training Agents to Learn to Ask for Help in Virtual Environments,” in which he will describe ongoing work in the space of assisted agent navigation where artificially intelligent (AI) agents can ask humans for help, and describe their own behaviors.
Additional workshops by CLIP members include:
Yang Trista Cao, a fourth-year computer science doctoral student, helped organize the Second Workshop on Trustworthy Natural Language Processing. Advancements in AI have historically been driven by the goal of improving model performance as measured by accuracy, but recently the NLP research community has started incorporating additional constraints to make sure models are fair and privacy-preserving. However, these constraints are not often considered together. The workshop aims to bring together these distinct yet closely related topics.
Philip Resnik, a professor of linguistics, will take part in the Eighth Workshop on Computational Linguistics and Clinical Psychology: Mental Health in the Face of Change, which he co-founded in 2014. Its mission is to bring together researchers in computational linguistics and NLP, who use computational methods to better understand human language, infer meaning and intention, and predict individuals’ characteristics and potential behavior, with mental health practitioners and researchers.
CLIP papers being presented at NAACL are:
“Deconstructing NLG Evaluation: Evaluation Practices, Assumptions, and Their Implications” by Hal Daumé III with researchers from Stanford University and Microsoft Research
The team investigates how people who research and develop natural language generation methods think about the evaluation of those methods. Through semi-structured interviews and a survey study, they uncover practices and constraints that shape the evaluation of language generation methods, and the implicated ethical considerations.
“Theory-Grounded Measurement of U.S. Social Stereotypes in English Language Models” by UMD researchers Yang Trista Cao, Anna Sotnikova, Hal Daumé III, Rachel Rudinger and Linda Zou
The paper proposes to adapt models from social psychology to measure stereotypes within language models through word association tests. With this new setting, the researchers analyze how stereotypes in language models correlate with human stereotypes. They also explore how language models stereotype social groups with intersectional identities.
“Recognition of They/Them as Singular Personal Pronouns in Coreference Resolution” by UMD researchers Connor Baumler and Rachel Rudinger
Baumler and Rudinger propose a method to test coreference resolution systems’ ability to differentiate singular and plural they/them pronouns. They found that existing systems are biased toward resolving “they” pronouns as plural, even when the correct resolution is clear to humans.
“Partial-input baselines show that NLI models can ignore context, but they don’t” by UMD researchers Neha Srikanth and Rachel Rudinger
Natural language inference (NLI) datasets have been shown to contain statistical biases (or artifacts), essentially providing models with an opportunity to “cheat” by ignoring context. The researchers find that despite the presence of these shortcuts in NLI datasets, models still learn to use all parts of an example to make a prediction, indicating that it is hasty to conclude that models trained on artifact-ridden datasets are not capable of reasoning.
“BitextEdit: Automatic Bitext Editing for Improved Low-Resource Machine Translation” by UMD’s Eleftheria Briakou and researchers from Facebook AI
Mined bitexts can contain imperfect translations that yield unreliable training signals for Neural Machine Translation. While filtering out such pairs is known to improve final model quality, the researchers argue that it is suboptimal in low-resource conditions where even mined data can be limited. They propose instead to refine the mined bitexts via automatic editing.
—Story by Melissa Brachfeld