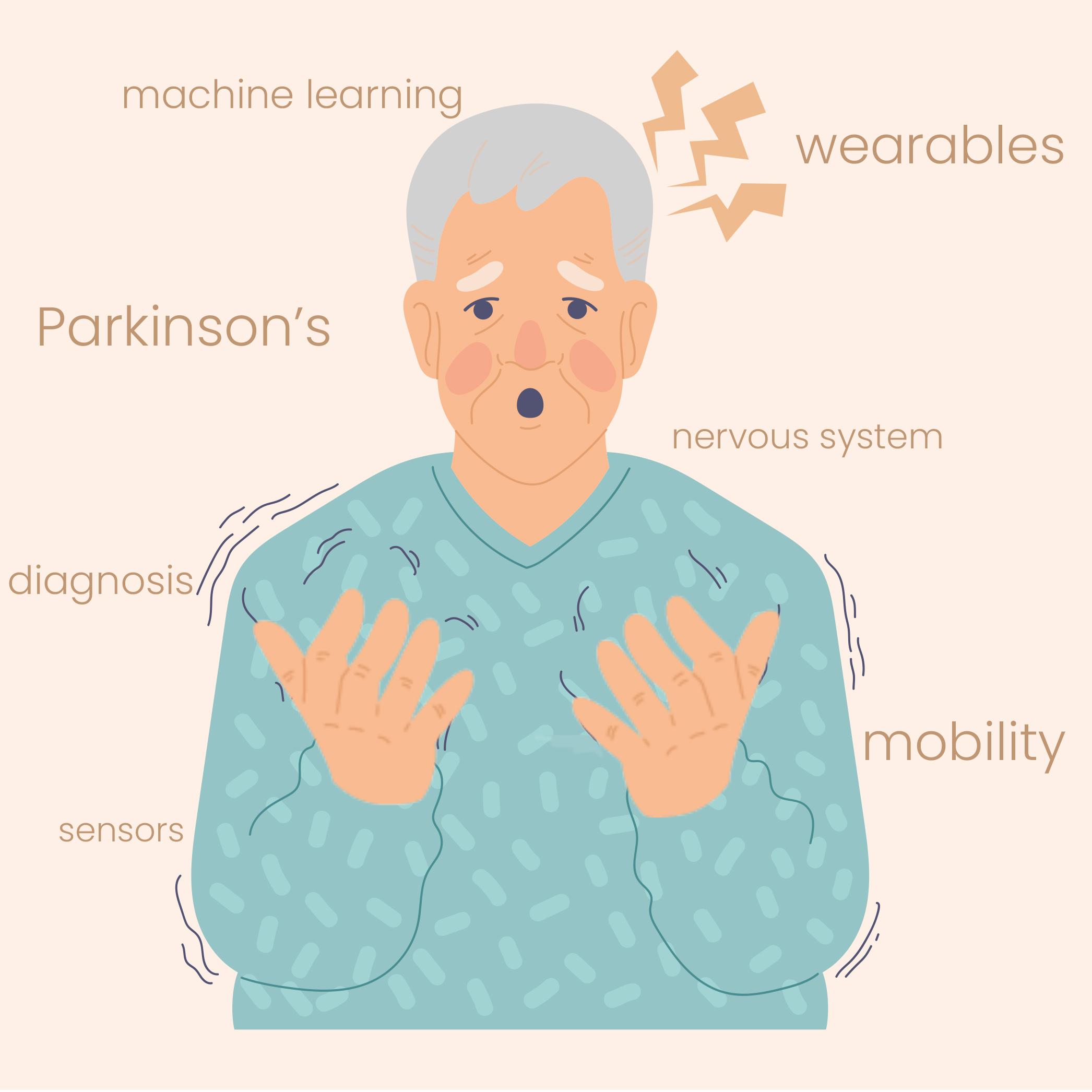
Researchers in the University of Maryland’s Center for Bioinformatics and Computational Biology (CBCB) have just published research that may soon help doctors better diagnosis patients with Parkinson’s disease.
Their work, done in tandem with researchers at the University of Maryland, Baltimore and others, uses machine learning algorithms to analyze data from wearable sensors that track movement. Ultimately, the researchers say, this can lead to more accurate and earlier diagnoses of people suffering from Parkinson’s, which in turn can lead to earlier therapeutic interventions.
Rana Khalil, a sixth-year Ph.D. student in computer science at UMD and lead author of the paper detailing the research, worked on the project with her adviser, Michael Cummings, a professor of biology who is the director of CBCB.
The project was funded by a $150,000 seed grant from the University of Maryland Strategic Partnership: MPowering the State, known as MPower, an initiative that fosters collaboration between the University of Maryland, College Park, and the University of Maryland, Baltimore.
Parkinson’s disease is a nervous system disorder that affects movement. It has a slow onset, starting with mild symptoms, such as a subtle tremor in one hand, that may progress to severe muscle rigidity and an inability to walk without help. According to the National Institutes of Health, approximately 500,000 people in the U.S. have been diagnosed with Parkinson’s.
But because many people are undiagnosed—or have been misdiagnosed—experts believe that as many as one million Americans may have the debilitating disease.
This considerable discrepancy highlights one of the central problems faced by clinicians and patients: the challenge of accurately diagnosing the disease. “The diagnosis of mobility disorders is very difficult,” explains Cummings. “Much of the process is subjective, and as such, isn’t highly accurate.”
As currently practiced, doctors diagnose patients with Parkinson’s by asking them to do a variety of mobility tasks, observing their walking and movement patterns, and testing their reflexes. However, this process is time-consuming and requires a lot of effort from both the clinician and the patient.
“We want to use objective measures to make the process more accurate and efficient,” says Cummings, who has an appointment in the University of Maryland Institute for Advanced Computer Studies (UMIACS). “By improving the time- and cost effectiveness of the process, we intend to benefit health care practice.”
If successful, he adds, this improvement would alleviate the physical and psychological distress patients face by cutting down on multiple clinic visits and misdiagnoses.
Although wearable sensors to diagnose Parkinson’s have been developed in the past, their complexity has prevented doctors from using them in clinics. The new research, published in MDPI’s journal Sensors, simplifies the use of sensors and machine learning in a clinical setting and makes it more practical, Cummings says. It demonstrated that a single sensor placed on the lower back and a single mobility task involving multiple movements can effectively distinguish individuals with Parkinson's disease from controls. The researchers then developed a sophisticated machine learning framework that analyzed patterns and variations in the data.
This enhanced the identification of disease symptoms and diagnostic accuracy of the disease, with the study reporting an accuracy of 92.6% in identifying participants across different Parkinson’s stages, surpassing the accuracy of 81% previously reported for clinical diagnosis by movement disorder experts.
This significant success was preceded by numerous difficulties, such as in data collection and signals processing. However, both Khalil and Cummings acknowledged that the computing resources from the CBCB and the financial support from the MPower initiative proved essential in overcoming these challenges.
“The MPower grant provided a reliable source of support for Rana,” Cummings says. “It enabled us to work on this project, gave us structure and a clear focus, and facilitated collaboration between our team and our partners.”
Moving forward, CBCB researchers are already deeply involved in a related study on distinguishing Parkinson’s from other movement disorders, attempting to further enhance accuracy and deter misdiagnosis.
—Story by Aleena Haroon, UMIACS communications group
Their work, done in tandem with researchers at the University of Maryland, Baltimore and others, uses machine learning algorithms to analyze data from wearable sensors that track movement. Ultimately, the researchers say, this can lead to more accurate and earlier diagnoses of people suffering from Parkinson’s, which in turn can lead to earlier therapeutic interventions.
Rana Khalil, a sixth-year Ph.D. student in computer science at UMD and lead author of the paper detailing the research, worked on the project with her adviser, Michael Cummings, a professor of biology who is the director of CBCB.
The project was funded by a $150,000 seed grant from the University of Maryland Strategic Partnership: MPowering the State, known as MPower, an initiative that fosters collaboration between the University of Maryland, College Park, and the University of Maryland, Baltimore.
Parkinson’s disease is a nervous system disorder that affects movement. It has a slow onset, starting with mild symptoms, such as a subtle tremor in one hand, that may progress to severe muscle rigidity and an inability to walk without help. According to the National Institutes of Health, approximately 500,000 people in the U.S. have been diagnosed with Parkinson’s.
But because many people are undiagnosed—or have been misdiagnosed—experts believe that as many as one million Americans may have the debilitating disease.
This considerable discrepancy highlights one of the central problems faced by clinicians and patients: the challenge of accurately diagnosing the disease. “The diagnosis of mobility disorders is very difficult,” explains Cummings. “Much of the process is subjective, and as such, isn’t highly accurate.”
As currently practiced, doctors diagnose patients with Parkinson’s by asking them to do a variety of mobility tasks, observing their walking and movement patterns, and testing their reflexes. However, this process is time-consuming and requires a lot of effort from both the clinician and the patient.
“We want to use objective measures to make the process more accurate and efficient,” says Cummings, who has an appointment in the University of Maryland Institute for Advanced Computer Studies (UMIACS). “By improving the time- and cost effectiveness of the process, we intend to benefit health care practice.”
If successful, he adds, this improvement would alleviate the physical and psychological distress patients face by cutting down on multiple clinic visits and misdiagnoses.
Although wearable sensors to diagnose Parkinson’s have been developed in the past, their complexity has prevented doctors from using them in clinics. The new research, published in MDPI’s journal Sensors, simplifies the use of sensors and machine learning in a clinical setting and makes it more practical, Cummings says. It demonstrated that a single sensor placed on the lower back and a single mobility task involving multiple movements can effectively distinguish individuals with Parkinson's disease from controls. The researchers then developed a sophisticated machine learning framework that analyzed patterns and variations in the data.
This enhanced the identification of disease symptoms and diagnostic accuracy of the disease, with the study reporting an accuracy of 92.6% in identifying participants across different Parkinson’s stages, surpassing the accuracy of 81% previously reported for clinical diagnosis by movement disorder experts.
This significant success was preceded by numerous difficulties, such as in data collection and signals processing. However, both Khalil and Cummings acknowledged that the computing resources from the CBCB and the financial support from the MPower initiative proved essential in overcoming these challenges.
“The MPower grant provided a reliable source of support for Rana,” Cummings says. “It enabled us to work on this project, gave us structure and a clear focus, and facilitated collaboration between our team and our partners.”
Moving forward, CBCB researchers are already deeply involved in a related study on distinguishing Parkinson’s from other movement disorders, attempting to further enhance accuracy and deter misdiagnosis.
—Story by Aleena Haroon, UMIACS communications group