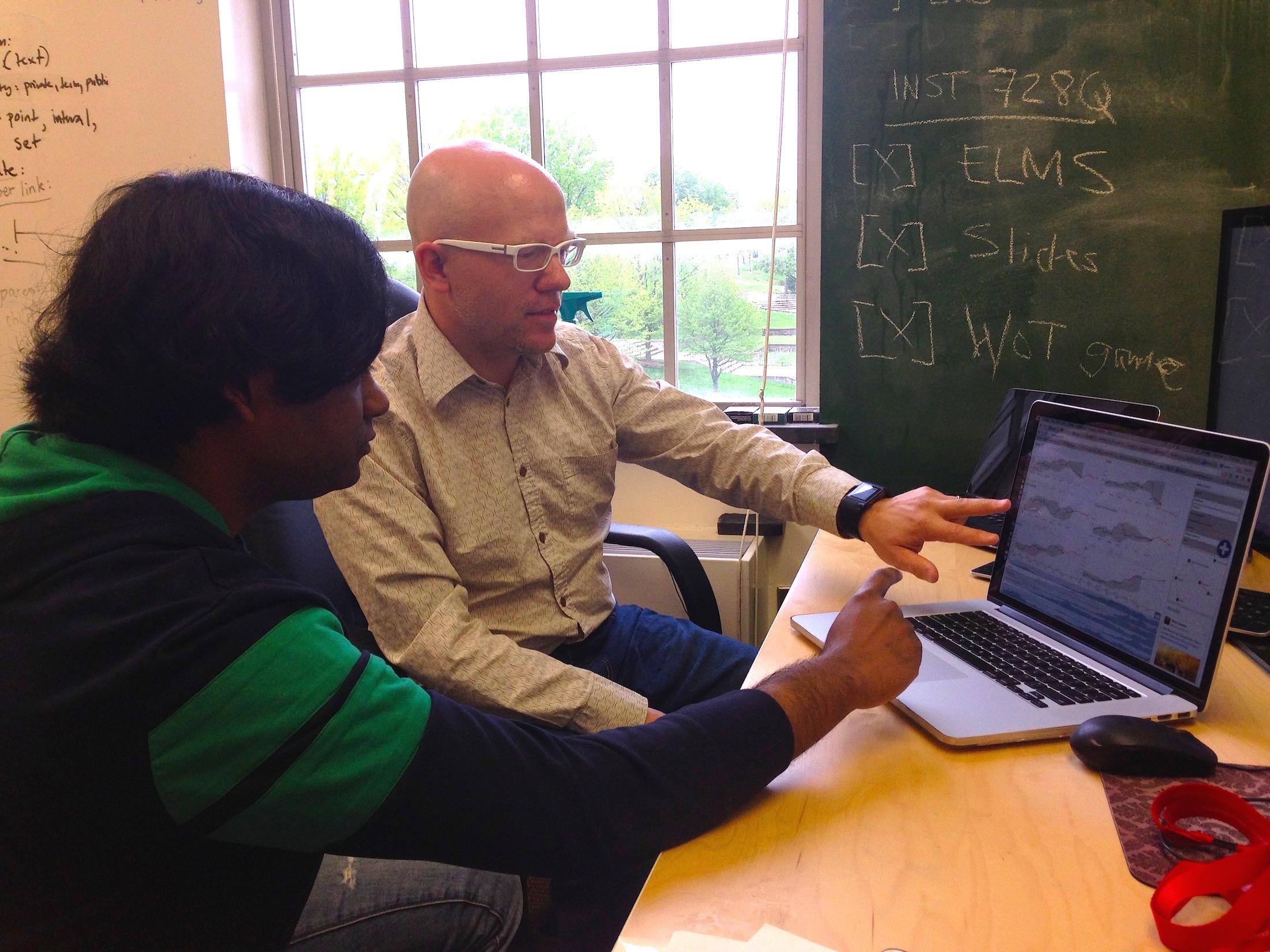
Modern computers are able to crunch massive amounts of information that changes over time, which has proven useful in areas like predicting economic trends, weather patterns and the spread of infectious diseases.
Looking to expand upon this model, a team of University of Maryland experts is adding human knowledge into the big data mix, making for better predictions that can ultimately lead to better outcomes.
The innovative technology and user interface—called TimeFork—is detailed in a paper presented this week at ACM CHI 2016, the top conference for human-computer interaction being held this year in San Jose, California.
“Big data is a very powerful tool, but it can’t predict everything. Human inference and observations are also needed,” says Niklas Elmqvist, an associate professor in the College of Information Studies (iSchool) and a member of the University of Maryland Institute for Advanced Computer Studies (UMIACS).
The key, Elmqvist says, is to accurately capture “user opinions”—a stock analyst’s take on what he reads in news articles or on social media, for example—and combine those valued opinions with massive amounts of raw data generated by computer algorithms.
TimeFork—a web-based interface with a sophisticated computational back-end—is applicable to any time-series dataset containing multiple variables likely to change over time, including financial markets (e.g., stocks), meteorological experiments (e.g., weather data), and medical practices (e.g., disease outbreak and treatment).
Accurate predictions are often quite challenging in these datasets, Elmqvist says, as they usually contain external factors that can affect future outcomes. Pending legislation on fracking, for example, can influence oil-related stocks. Or, international trade agreements can affect product manufacturing in certain countries or regions.
TimeFork provides a way to incorporate this type of peripheral information, which normally requires human interpretation, into computer-based prediction models.
Sriram Karthik Badam, a 3rd-year doctoral student in computer science who is the lead author of the paper, describes a hypothetical scenario using TimeFork: The computer might first show possible future trends of Apple and Samsung stocks; the user can then provide his/her opinion about the future (that Apple’s stock might increase due to an iPhone 7 release, for example); the computer then updates its own predictions for other variables such as Samsung's stock decreasing if Apple increases.
“We’re trying to stimulate a conversation between computers and humans—we want humans to become an active partner in the dialogue with these mathematical models,” Badam says, adding that early hypothetical testing of TimeFork on the U.S. stock market has shown promising results.
The researchers say that these types of interactive systems—where humans and machines work toward solving complex problems together—will inspire the next generation of user interfaces.
Badam and Elmqvist are doing the bulk of their research in the university’s Human-Computer Interaction Laboratory, which is jointly supported by the iSchool and UMIACS. They are collaborating on the project with Jieqiong Zhao and David Ebert from Purdue University and Shivalik Sen from the Birla Institute of Technology and Science in India.
Elmqvist would like to see systems like TimeFork used for many complex decisions that deal with time-changing data, including energy conservation or health-related informatics.
“Our results, while preliminary, provide an important evidence of the efficacy of interactive systems in data science,” he says.